Brian Hanking, CTO • Canara
Data centers are the hard drives of the Internet. In fact, Wikibon asserts them as “the center of modern software technology, serving a critical role in the expanding capabilities for enterprises.” Despite their importance, they are still victim of unplanned downtime, the majority of which can be attributed to battery failure of some kind. According to the Ponemon Institute, 91 percent of data centers have experienced an unplanned outage in the past 24 months, the cost of which rose from $5.6K a minute in 2010 to $7.9K a minute in 2013. Today, in 2016, the cost is thought to have doubled with the mounting demands on data centers and their ever-expanding reach through cloud and virtual technologies. As organizations increasingly rely on data centers to support their business operations, and those critical facilities rely on battery rooms and backup power infrastructure, the data center industry must change its mindset from reactively responding to downtime to proactively preventing it.
“This increase in cost underscores the importance for organizations to make it a priority to minimize the risk of downtime that can potentially cost thousands of dollars per minute,” said Larry Ponemon, Ph.D., chairman and founder, the Ponemon Institute.
Over the past few decades, we have in fact seen a positive shift across the data center industry’s operations strategy from a “fixing it when it breaks” mentality to physically monitoring the equipment in real-time through the use of sensors attached to just about every piece of technology in the data center. Today, data center operators often take manual readings on all the equipment every day and input those readings into a spreadsheet, viewing them for about a month before discarding them. Even locally monitored battery systems produce thousands of points of data every day, however data in itself is not knowledge, and monitors do not have the capability to generate any actionable insight without proper in depth analysis with reference to past readings.
What if this labor-intensive analysis was automated with the proper logic? What if data center operators had the ability to compare their readings to the historical data from, not just their own equipment, but that brand of equipment nationally or even internationally? While monitoring assets on a daily basis is a great start in terms of optimization strategy, operators should go one step further and analyze and compare it with the past data collected to actually predict a failure and prevent any damages through pattern recognition and in depth analysis. It’s a simple last step that many fail to take.
Let’s take a look at the evolution of the data center, the increasingly vital need for more efficient utilization of resources, and how a shift in operations strategy from reactive to proactive through predictive analytics can improve performance, prolong asset life and maximize ROI.
The 1980s: The “Fix It When It Breaks” Mentality
Though its origins technically began in the late ‘60s with the development of ARPANET, the Internet was still considered in its infancy in the ‘80s. In 1981, IBM introduced personal computers to the world and then portable computers a few years later, which quickly resulted in the computer industry boom, as companies saw a significant benefit in deploying desktop computers throughout their environment. With more and more computers being installed, information technology (IT) operations started to grow in complexity.
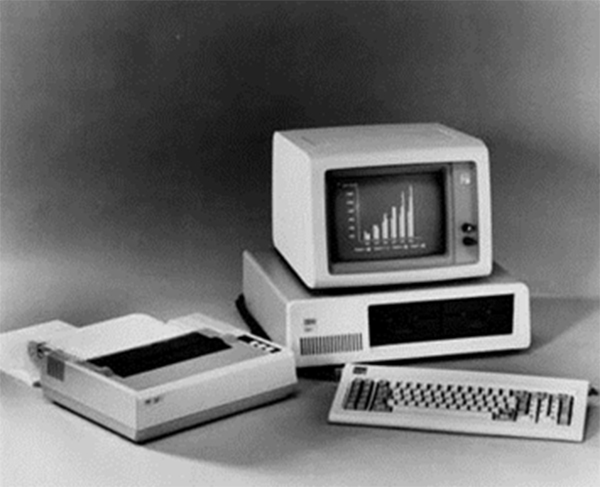
In the data center industry, however, the majority of equipment was isolated and basic, with the common operations strategy being reactionary. In other words, data center operators would simply fix equipment when it breaks, and only perform routine maintenance about once a quarter.
In terms of batteries, companies began to shift from wet cell to VRLA (value-regulated lead-acid) technology. This trend started in the ‘80s, with the new “maintenance free” VRLA batteries, footprints tended to be smaller and more convenient, and cell watering was no longer required with VRLA having a sealed internal cycle. The US, in particular, quickly adopted the almost cassette-like technology as data center growth bloomed. While everything was fine for the first couple of years following the introduction of VRLA, it soon became evident that “maintenance free” was perhaps the wrong term and “low maintenance” would have been the more appropriate phrase. The fact that no one could see inside the cells meant that monitoring these batteries became a prerequisite.
The 1990s: Dot-Com and The Modern Data Center
As we have witnessed throughout the history of tech innovations, massive consumer adoption eventually leads to business implementation. The ‘90s was no different, as the Internet reached mass-market adoption by the middle of the decade.
The birth and subsequent boom of the modern data center took place during this dot-com era, as well, which replaced the mainframes in computer rooms with microcomputers (servers) within companies’ own offices. The software itself increased in complexity; as organizations demanded permanent Internet presence, network connectivity and colocation. Despite an increasingly modern environment, the backup power system of a critical facility was, and is still, completely dependent on room full of batteries. Unlike the preceding decade, routine maintenance began to incorporate reporting, this time performed by more “machine specialists” then the jack-of-all-trade mechanics of the ‘80s. These specialists will continue to become more necessary well into the 2000s as more complex technology is added to legacy equipment.
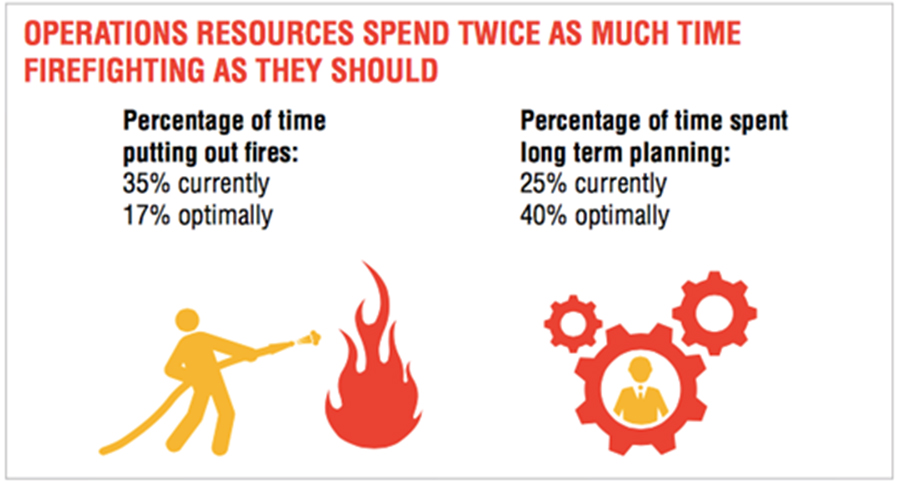
The 2000s: A Booming Industry
By Y2K, every company had an online presence, with electronics and computers being used in equipment to improve efficiency and reduce costs. In addition, equipment started to be put on the IT network and building management became a focal point in the data center. By 2002, data centers were responsible for 1.5 percent of the total US power consumption, a number that has increased by 10 percent every year since.
In the second decade of the 2000s, many companies transition to having only an Internet presence as a result of the virtual technology revolution. In fact, according to Wikibon, by 2011, 72 percent of organizations said their data centers were at least 25 percent virtual. No longer isolated and siloed, data centers are able to create a centralized environment through advanced technology. While a benefit to productivity and efficiency, the addition of new, complex technology brings with it more opportunities for failure, the majority of which is still attributed to battery failure of some kind. It only takes one single unit failure within a string of batteries to render the entire string useless. Therefore, only a few bad units can result in the failure of the entire emergency power system. This only reinforces the need for specialized engineers that are focused on monitoring and analyzing batteries in order to prevent downtime by identifying problems and predicting failure.
Today, nearly every piece of equipment in a data center has a sensor attached to it, and monitoring systems have the autonomy to alert operators in the event of any abnormality, collecting and storing data throughout the asset’s lifecycle. Currently, however, no battery monitoring system is intelligent enough to actually analyze the data, so to extract any practical insight someone must look at all the data in which the system provides. Admittedly, this is quite a labor-intensive task that requires a specific skillset that, as a result, rarely is performed by a data center operator. The result is a lack of visibility into the battery room and backup power infrastructure.
Instead of empowering operators with the confidence to prevent incidents and outages, monitoring systems actually create a false sense of security. The operations team gets locked into a passive role, assured that the monitoring systems will alert them if there is an issue. However, by the time the equipment alarms an operator, it is far too late to take any action. The damage is already done and the staff is forced into reaction mode. The Uptime Institute supports this notion, recently revealing in their 2015 Data Center Industry Survey that operations staff spends twice as much time putting out fires than they should, such as responding to temperature and AC alarm issues. As a result, little time is available for strategic and proactive planning, despite the overwhelming need for a better solution.
While deploying a monitoring system is certainly a step in the right direction, increasing costs of downtime paired with the technical complexity of data center equipment requires operators to go one step further to obtain any meaningful results.
Meeting the Demands of the Data Center
The proliferation of the Internet of Things (IoT) and increasingly dynamic environments are placing profoundly more demands on the data center. As such, data center operators are facing mounting pressure to manage their facilities more efficiently and cost effectively while still achieving 100 percent uptime. Yet, this is proving to be a difficult obstacle to overcome, with energy being the fastest growing component of data center OpEx. In fact, according to Wikibon, “a major challenge in IT today is that organizations can easily spend 70 percent to 80 percent of their budgets on operations including optimizing, maintaining and manipulating the environment.”
In an effort to conquer this challenge, data center operators are deploying virtualized servers, consolidating facilities and managing capacity with actual demand. Yet, remarkably, they blindly replace full strings of batteries, averaging $12,000.00 each, typically every four years without a second thought – resulting in significant financial losses or unnecessary investments if either 1) the string of batteries fails in less than four years and causes an outage or 2) the entire string of batteries is replaced prematurely, not utilizing the assets to their actual useful life.
According to Ponemon Institue, the most common cause of data center downtime is uninterruptible power supply (UPS) failure, and an estimated 85 percent of UPS incidents are due to battery failure. However, the responsibility for looking at this vital piece of equipment is often given to someone who has several other roles within the facility and someone who is not an expert. Therefore, batteries are often overlooked and when an issue does come up, it is inevitably too rapid and too late to address it before the power drops out.
Although small in stature, batteries play a critical role in data center operations. But without proper analysis, facility operators do not have insight into the status of their batteries and rely only on quarterly maintenance and regular replacements. By exploring current and historical data, however, we have the ability to improve performance, prolong asset life and maximize ROI through prediction and prevention.
Maximizing ROI with Predictive Analytics
In 451 Research’s Voice of the Enterprise Q1 2015 report, IT professionals state that their top three priorities for the enterprise are:
• Increased IT asset utilization;
• Data center consolidation;
• Alignment of data center and business processes.
There is a clear disconnect between the priorities of the executives and the performance of the operations staff. The IT budget allocated to data center operations is declining and pressure for efficiency is climbing, yet operators still spend nearly half their time and resources putting out costly fires. In addition, operators are relying on industry standards and blindly replacing assets that, dependent on numerous factors, have either already failed or not yet reached their maximum life. This not only puts a dent in equipment budgets, but also human capital.
To prevent such expenditures and appease both parties, there needs to be a cultural shift in the data center industry from responding to a failure to predicting one long before it becomes an alarm. With nearly every piece of equipment connected to a sensor, the opportunity to do so is already available. Specifically for batteries, applying predictive analytics ensures power continuity and allows operators to budget more effectively, ultimately protecting their investment. Yet in this modern age, we often get so immersed in the fact that we can gather data from almost anything, that we forget about what we are going to do with that data when it is collected.
So how can we turn all of this data into actionable insights? There are five steps.
1. Record the data
2. Compare the data
3. Identify patterns
4. Apply experience
5. Make decisions
With the first step already accomplished, data center operators have the opportunity to then look at the data and compare it to historical data collected. Instead of relying on generic industry standards or blindly replacing equipment every few years, data center operators are empowered with data collected from the exact same brand of asset to identify patterns and apply past experience to their current situation in order to make informed decisions.
In order to retrieve value and actionable insight, the data must be analyzed with proper logic and analysis in order to identify problems before it’s too late. By constantly reading, recording and scanning equipment, one can identify rising or falling trends that indicate impending problems as well as identify assets that have reached end of life. In addition to maintaining uptime and correct operation when needed, proper monitoring and analysis can guarantee that the maximum life is extracted from the battery investment by ensuring that it is taken care of properly. To maximize allocation of resources, hand the information over to the experts just like you do with your security team for building access, allowing you to focus on more productive responsibilities, confident that your assets are taken care of. This is perhaps the most efficient use of a monitoring system, as expert can look at the data every day and use predictive analytics to provide earlier warning of possible issues, more assured uptime, elimination of false alarms, flagging of additional issues, extending useful battery life, enabling better asset management and assistance with warranty claims.
For example, a battery monitoring specialist would set the baseline for the assets’ aging characteristics. If the battery is new then it is simply a matter of waiting for the values to settle and storing them. If the battery is several years old, however, experts can use previous battery signatures to determine what the values for that system would have been when new, which allows them to accurately forecast the correct end of life. In addition, an added benefit of monitoring batteries is the ability to identify indications that other equipment may be failing, such as when a capacitor is leaking and an SCR board had failed. This information is then presented to the data center operator as a report, who is then empowered with the knowledge of the true health of the assets and the confidence to present the findings to senior executives.
There is no avoiding the fact that batteries fail, but monitoring and predictive analytics can have a dramatic effect on identifying warning signs early and eliminating problems before they occur. In addition, looking back at historical data helps set a baseline to look forward, ensuring uptime, prolonging asset life and maximizing ROI.
For more information, visit Canara at http://canara.com.